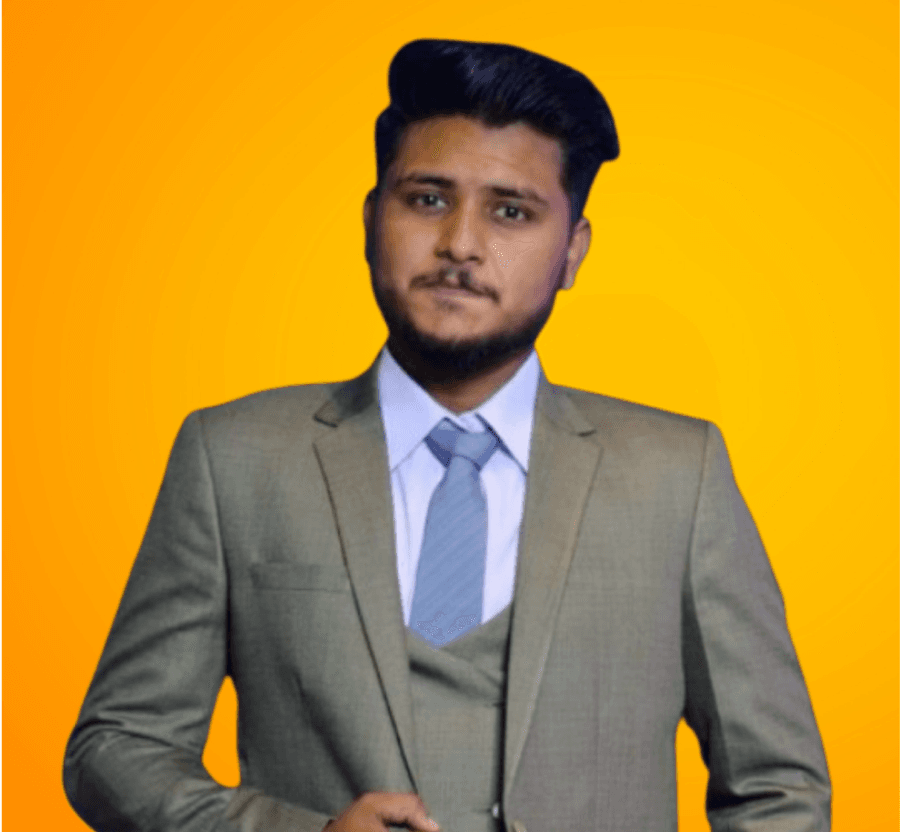
Usama Shahid
Head of Marketing and Development




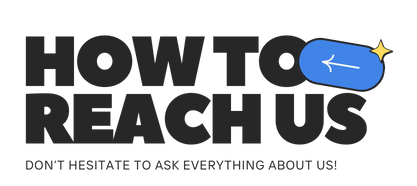
Get In Touch With Our Team
Related Blog:
Let get started.
Ready to turn your app idea into reality? Get in touch with us today!
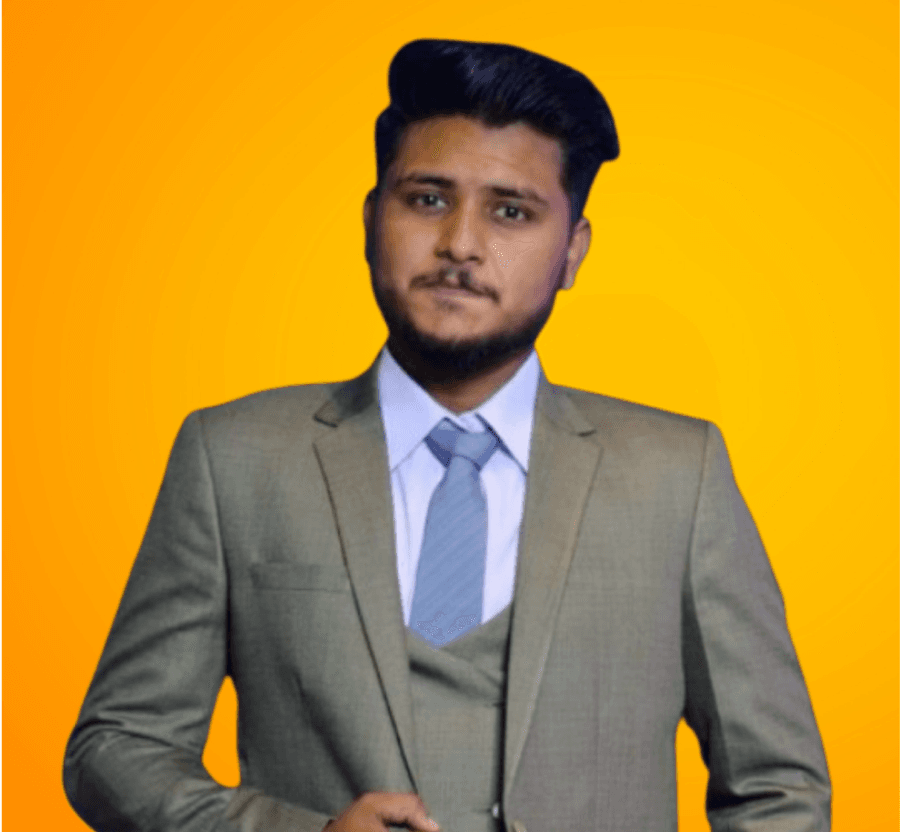
Usama Shahid
Head of Marketing and Development
"We can make your digital dreams a reality in the United States with our top-tier services, allowing you to experience the seamless integration of technology. We offer tailored services to meet your specific needs under one roof since we understand how important your digital footprint is in today's competitive world of technology. To give you the best service possible, our expert team is driven by a love for innovative ideas."
Call us at,
Send Enquiry at,